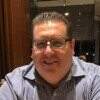
Vantage Social Network Analysis Framework for Covid-19 Risk Metrics
Vantage Social Network Analysis (SNA) Framework helps us with useful insights to identify various risk factors associated with the spread of Covid-19. Read more.

Social Network Analysis (SNA) is the process of exploring network structures according to graph theory. Based on human interactions, SNA helps us to establish different kinds of relationships similar to the social network. By systematically analysing the data on these relationships, it is possible to establish deeper insights into the behaviour of people in accordance with their role in the network. SNA analytics provide us with crucial metrics to analyse the risk associated with the spread of Covid-19 among the individuals and communities in a geographical location.
SNA Network
The below Vantage visualization represents a SNA network. Each node in the SNA graph represents a person or a tracking device while the edges represent the connection made by the nodes in the network.
Risk of Introduction (Betweenness)
These people reside in the periphery of several large communities and have the potential to “Introduce” the virus to the entire network of communities.
Example: We have an asymptomatic person coming into a city via the airport and taking a metro to reach home. He has not travelled extensively in the city; however, he has introduced the virus in key places like “Metro Stations.”

Risk of Spread (Closeness)
High closeness centrality individuals tend to be “Agents of Spread” within their local network community.
Example: A pizza delivery guy traveling extensively within a small community of people.

Risk of Danger – Eigenvector Centrality
Individuals with high eigenvector centrality scores are often more likely to be more “Agents of High Vulnerability” to the infection spread.
Example: These are the front line medical workers getting exposed to people with infection.

Individual Risk Scores

Community Risk

Clustering Coefficients give us an idea about the structure of the graph rather than the importance of the nodes themselves.

Global Clustering Coefficient measure gives an indication of the clustering in the whole of network. It is a ratio of the number of closed triplets formed to possible number of triplets that can be formed within a network.
Global clustering coefficient helps us to compare the risk associated with a community of people in a locality with other localities.
Example: We can compare the risk associated with a particular floor with the other floors in the building.
Local Clustering Coefficient is a measure of embeddedness of the individual in the cluster. The average of local clustering coefficient gives us an indication of the behavior of the individual nodes in the community.

Enhanced Contact Tracing:
Traditional Tracking

Enhanced Tracing

Reverse Contact Tracing

Drill Down Tracing

Popular Pattern of Movement

In conclusion, with the help of Teradata Vantage’s advanced analytic functions, the SNA analytics framework helps us with useful insights to detect patterns of life and identify various risk factors associated with the spread of Covid-19.
知っている人に滞在
Teradataのブログを購読して、毎週あなたに配信されるインサイトを入手する